As I mentioned above, the two primary use cases for using predictive analytics in marketing is for qualification and prioritization of demand. If you know what leads have the highest likelihood to convert, you can use that score and implement a threshold to limit the amount of unqualified leads getting to your sales floor. It’s also likely that you’ll find leads you aren’t currently passing on to your sales team that could be assigned. In order to maximize the effectiveness of those leads, you’ll also want to use some sort of view for Sales that prioritizes the leads by score. I like to say that if your reps can make 80 phone calls in a day and you’re sending them 100 leads, you better be sure that those 80 calls are going to the right prospects. Using a predictive score to help prioritize can make your sales reps
Another method to unlock more value is to have your medium or low-rated leads go to a Sales Development team to do manual qualification on. If certain pockets of these leads convert at a decent clip, the next iteration of your model refresh should value them appropriately. This will make sure the right resources in sales are matched to the right set of activities to achieve the highest level of productivity.
Regression analysis in its various forms is the primary tool that organizations use for predictive analytics. It works like this in general: An analyst hypothesizes that a set of independent variables (say, gender, income, visits to a website) are statistically correlated with the purchase of a product for a sample of customers. The analyst performs a regression analysis to see just how correlated each variable is; this usually requires some iteration to find the right combination of variables and the best model. Let’s say that the analyst succeeds and finds that each variable in the model is important in explaining the product purchase, and together the variables explain a lot of variation in the product’s sales. Using that regression equation, the analyst can then use the regression coefficients—the degree to which each variable affects the purchase behavior—to create a score predicting the likelihood of the purchase.
If you’ve bought into predictive analytics for marketing conceptually, then you’ll need to begin your vendor search. This is a fairly crowded market these days and I have not personally used each and every platform below. Based on what I’ve heard from other marketers, these are the top players in the space:
- Infer: the solution I have the most familiarity with, Infer bills itself as a Predictive Sales and Marketing platform. It was fairly straightforward to implement – we just had to get them proper levels of access to our CRM, provided some rough guidance in how we wanted to use them, and they create the predictive model based on our data and began scoring leads at regular intervals. They are also adding the capability to add in net new contacts that fit your profile.
- 6Sense: they differentiate their predictive solution through ‘uncover[ing] net-new prospects at every stage of the funnel’ and focus more on larger B2B organizations. They were born out of a custom predictive analytics project at Cisco and count Dropbox, ADP, and Netsuite as customers. Their founder, Amanda Kahlow, also has a very interesting story in how they came to bring on Salesforce as an investor.
- Lattice Engines: also focused more on larger customers, Lattice Engines positions itself as a part of Account-Based Marketing, scoring and helping prioritize accounts for your marketing and sales teams. Dell, Citrix, and Staples are on their customer list.
- Everstring: ‘leverages data to predict your next best customer.’ They call themselves a decision platform and seem more focused on the sales side of the equation compared to their peers, specifically calling out how sales development and sales reps can use the platform.
I’ve had many marketers ask me for my opinions about different predictive analytics providers. I’ll tell you what I often tell them – from my experience, you aren’t going to find much differentiation in the models themselves. They all use the same mathematical techniques to create the predictive models, using similar data sources so even in a typical bake-off it’s not likely that one provider’s model would be really good and another would be mediocre. Thus when you are conducting your evaluation I believe the most important factor is whether you like the organization and the product. If you have a good trial experience and the price matches the perceived value, I would move forward and feel comfortable with it.
There is another option you can consider, and roll your own solution. I’ve used this option in the past when predictive as a service wasn’t as developed. If you have access to the right kind of data and the capability internally, it’s a viable option. There are consultants who also specialize in this as well, but I think given the plethora of options out there, most organizations are better off using one of the companies above.
Besides the obvious use cases for advanced lead scoring, almost all of the predictive analytics solutions out there are pushing to add capabilities to build target account lists and generate additional demand by either buying names at sets of target accounts, or highlighting existing demand at certain accounts. In the future, I believe these solutions will partner with account-based marketing technologies like DemandBase and Engagio to find the ‘next’ set of accounts that you should be focused on at your organization. Otherwise, they risk just being an expensive lead-scoring program. I’ll touch on this below when I talk about what’s next.
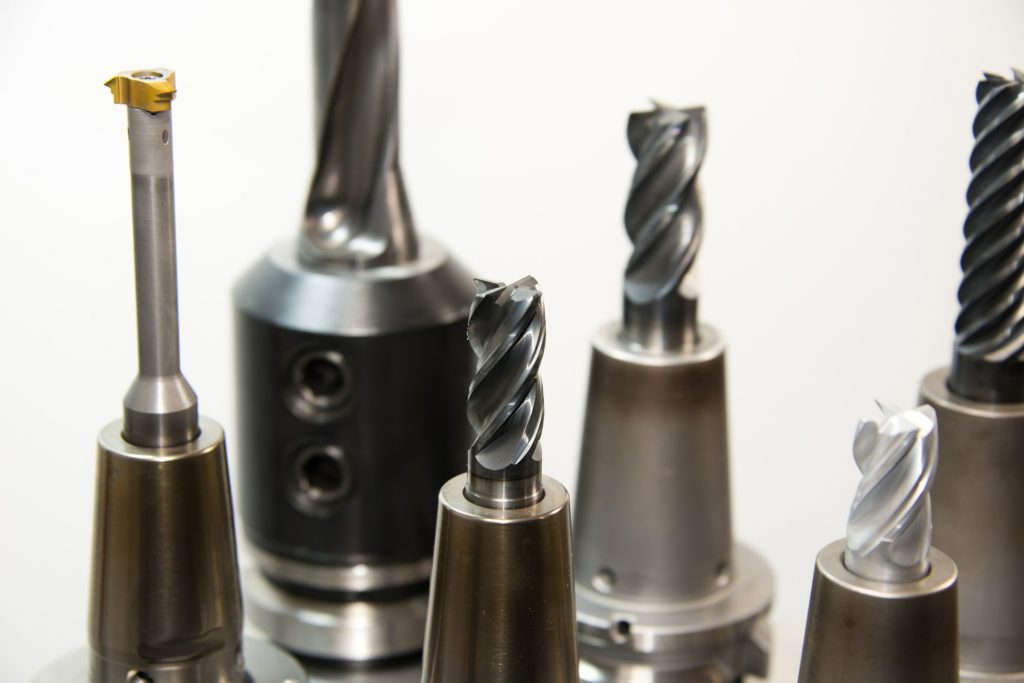
Best Practices in Applying Predictive Analytics for Marketing
- Make sure you get regular model refreshes as part of your agreement with any vendor (at least 2x/year), and that you enable it from your side by providing the right set of resources and access to appropriate systems so this is as seamless as possible.
- When you implement the scoring model, don’t make the scores immediately visible to front-line sales reps. Rather have it running in the background for the length of at least one sales cycle (or if your sales cycle is too long to make this practical, try doing this for long enough that you feel confident in the evaluation of a given cohort of leads). This kind of single-blind study will allow you to see how the model performs without changing Sales behavior.
- Get Sales leadership involved early, so they are bought in to the concept, and regularly update them on the progress of the project and implementation. If you learn something interesting during the blind rollout, share that with them.
- Use other methods of scoring or categorization to complement the vendor’s score. This is really important when going after new markets and buyers, or releasing new products. You may for instance want to segment portions of your audience and adjust scoring tiers as new types of buyers enter your marketing/sales funnel, because the scoring model in place might not see them as valuable until deals start to happen.
- Make sure you have regular catch-up calls and discuss new buyer personas and products (or any other changes in your Go To Market motions) with the vendors you work with. They want to make sure you are engaged and happy so it’s best to provide as much info as you can to them so they can set you up for success.
- Be proactive in thinking about how predictive analytics solutions might complement other technologies in your Marketing Technology stack – look at what ABM providers are offering for instance to identify possible overlaps and where you might be able to get the most value out of using them together. Or if there’s two much overlap, perhaps you don’t need different sets of technologies to meet your needs.
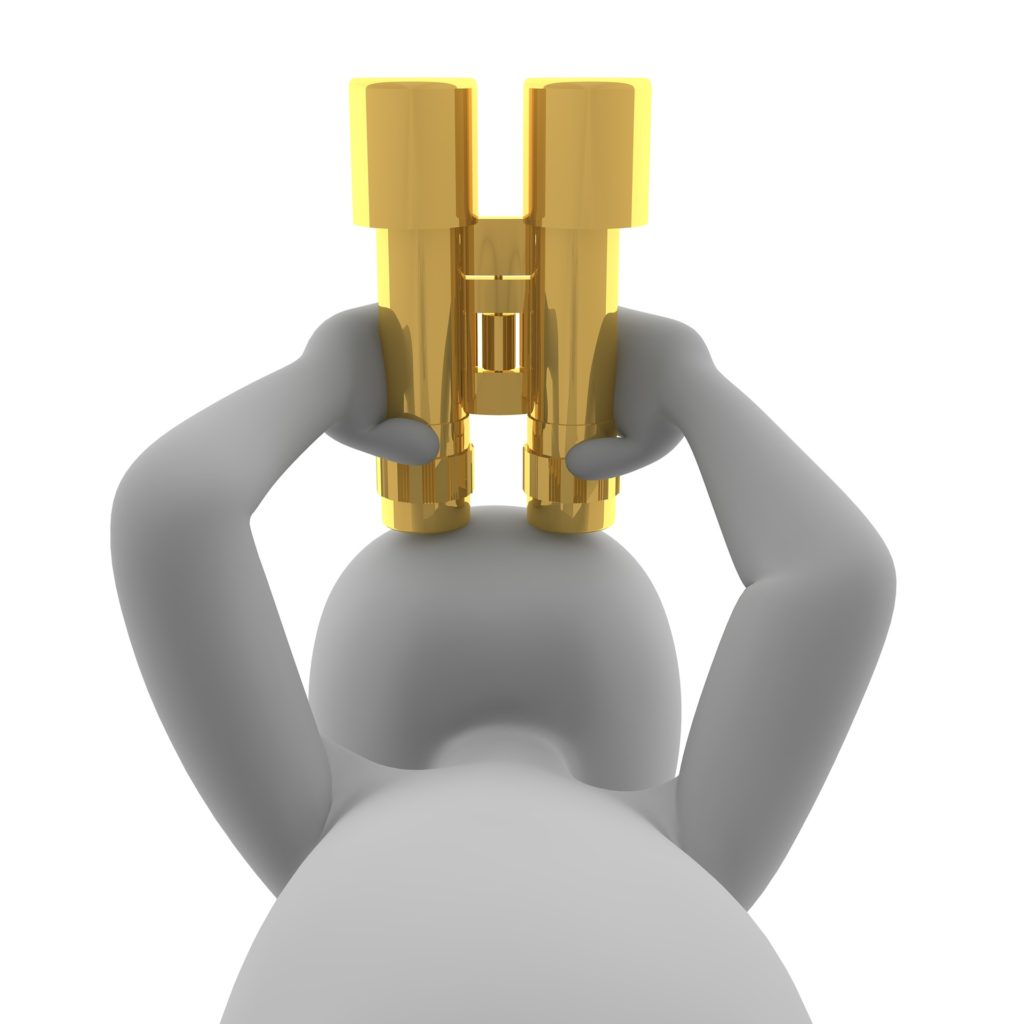